Do you know if your company’s data is clean and well managed? Why does that matter anyway?
Without a working governance plan, you might not have a company to worry about — data-wise.
Data governance is a collection of practices and processes establishing the rules, policies, and procedures that ensure data accuracy, quality, reliability, and security. It ensures the formal management of data assets within an organization.
Everyone in business understands the need to have and use clean data. But ensuring that it is clean and usable is a big challenge, according to David Kolinek, vice president of product management at Ataccama.
That challenge is even greater when business users must rely on scarce technical resources. Often, no one person oversees data governance, or that individual lacks a complete understanding of how the data will be used and how to clean it.
This is where Ataccama comes into play. The company’s mission provides a solution that even people without technical knowledge, such as SQL skills, can use to find the data they need, evaluate its quality, understand how to fix any issues, and determine whether that data will serve their purposes.
“With Ataccama, business users don’t need to involve IT to manage, access, and clean their data,” Kolinek told TechNewsWorld.
Keeping Users in Mind
Ataccama was founded in 2007 and basically bootstrapped.
It started as a part of Adastra, a consulting company, which is still in business today. However, Ataccama’s was focused on software rather than consulting. So management spun off that operation as a product company that addresses data quality issues.
Ataccama started with a basic approach — an engine that performed basic data cleansing and transformation. But this still required an expert user because of the user-provided configuration.
“So, we added a visual presentation for the steps that enable data transformation and things like cleansing. This made it a low-code platform since the users were able to do the majority of the work just by using the application user interface. But it was still a thick-client platform,” Kolinek explained.
The current version, however, is designed with a non-technical user in mind. The software includes a thin client, a focus on automation, and an easy-to-use interface.
“But what really stands out is the user experience, which is built off the seamless integration we were able to achieve with the 13th version of our engine. It delivers robust performance that’s tuned to perfection,” he offered.
Digging Deeper Into Data Management Issues
I asked Kolinek to discuss the data governance and quality issues further. Here is our conversation.
TechNewsWorld: How does Ataccama’s concept of centralizing or consolidating data management differ from other cloud systems such as Microsoft, Salesforce, AWS, and Google Cloud?
David Kolinek: We are platform agnostic and do not target one specific technology. Microsoft and AWS have their own native solutions that work well, but only within their own infrastructure. Our portfolio is wide open so it can serve all the use cases that must be covered across any infrastructure.
Further, we have data processing capabilities that not all cloud providers possess. Metadata is useful for automated processing, generating more metadata, which in turn can be used for additional analytics.
We developed both of these technologies in-house so we can provide native integration. As a result, we can deliver a superior user experience and a whole lot of automation.
How is this concept different from the notion of standardization of data?
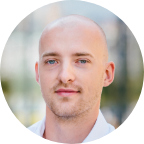
VP of Product Management,
Ataccama
Kolinek: Standardization is just one of many things we do. Usually, standardization can be easily automated, the same way we can automate cleansing or data enrichment. We also provide manual data correction when solving some issues, like a missing social security number.
We cannot generate the SSN, but we could come up with a date of birth from other information. So, standardization is not different. It is a subset of things that improve quality. But for us, it is not only about data standardization. It is about having good quality data so information can be properly leveraged.
How does Ataccama’s data management platform benefit users?
Kolinek: The user experience is really our biggest benefit, and the platform is ideal for handling multiple personas. Companies need to enable both business users and IT people when it comes to data management. That requires a solution for business and IT to collaborate.
Another enormous benefit of our platform is the strong synergy between data processing and metadata management it provides.
The majority of other data management vendors cover only one of these areas. We also use machine learning and a rules-based approach and validation/standardization, which, again, are often not both supported by other vendors.
Also, because we are technology agnostic, users can connect to many different technologies from the same platform. With edge processing, for instance, you can configure something once in Ataccama ONE, and the platform will translate it for different platforms.
Does Ataccama’s platform lock-in users the way proprietary software often does?
Kolinek: We developed all the core components of the platform ourselves. They are tightly integrated together. There has been a huge wave of acquisitions lately in this space, with big vendors buying smaller ones to fill in gaps. In some cases, you are not really buying and managing one platform, but many.
With Ataccama, you can purchase just one module, like data quality/standardization, and later expand to others, such as master data management (MDM). It all works together seamlessly. Just activate our modules as you need them. This makes it easy for customers to start small and expand when the time is right.
Why is a unified data platform so important in this process?
Kolinek: The biggest benefit of a unified platform is that companies are not looking for a point solution to solve just a single problem, like data standardization. It is all interconnected.
For instance, to standardize you must validate the quality of the data, and for that, you must first find and catalog it. If you have an issue, even though it may look like a discrete problem, it more than likely involves many other aspects of data management.
The beauty of a unified platform is that in most use cases, you have one solution with native integration, and you can start using other modules.
What role do AI and ML play today in data governance, data quality, and master data management? How is it changing the process?
Kolinek: Machine learning enables customers to be more proactive. Previously, you would identify and report an issue. Someone would have to investigate what went awry and see if there was something wrong with the data. Then you would create a rule for data quality to prevent a recurrence. That is all reactive and is based on something breaking down, being found, reported, and then fixed.
Again, ML lets you be proactive. You give it training data instead of rules. The platform then detects differences in patterns and identifies anomalies to alert you before you even realized there was an issue. This is not possible with a rules-based approach, and it is much easier to scale if you have huge amounts of data sources. The more data you have, the better the training and its accuracy will be.
Other than cost savings, what benefits can enterprises gain through consolidating their data repositories? For instance, does it improve security, CX outcomes, etc.?
Kolinek: It does improve security and mitigates potential future leaks. For example, we had customers who were storing data that no one was using. In many cases, they did not even know the data existed! Now, they are not only unifying their technology stack, but they can also see all the stored data.
Onboarding new people onto the platform is also much easier with consolidated data. The more transparent the environment, the sooner people can use it and start gaining value.
It is not so much about saving money as it is about leveraging all your data to generate a competitive advantage and generate additional revenue. It provides data scientists with the means to build things that will advance the business.
What are the steps in adopting a data management platform?
Kolinek: Begin with the initial analysis. Focus on the biggest issues the company wants to tackle and select the platform modules to address them. Defining goals is key at this stage. What KPIs do you want to target? What level of ID do you want to achieve? These are questions you need to ask.
Next, you need a champion to advance execution and identify the main stakeholders who could drive the initiative. That requires extensive communications among different stakeholders, so it is vital to have someone focused on educating others about the benefits and helping teams onboard the system. Then comes the implementation phase where you address the key issues identified in the analysis, followed by rollout.
Finally, think about the next set of issues that need to be addressed, and if needed, enable additional modules in the platform to achieve those goals. The worst thing to do is purchase a tool and provide it, but offer no service, education, or support. This will ensure that adoption will be low. Education, support, and service are very important for the adoption phase.
Social Media
See all Social Media